A new type of artificial nanowire neuromorphic network that can like a brain
- Chen Roc
- Jul 5, 2021
- 7 min read
By keeping the nanowire network in a state similar to that of the brain at the "edge of chaos", the system can perform tasks at an optimal level.
As we all know, the complexity of the human brain information processing system is unmatched by the current most advanced supercomputers. Tens of billions of neurons in the brain are connected through synapses to form a complex neural network. Under the complex interaction between the nonlinear elements of the nerve synapses and the cyclic network topology, the brain can achieve unique adaptive real-time learning.
In recent years, inspired by the brain's nervous system, neuromorphic computing has become an important research direction in the field of artificial intelligence. Scientists hope to develop computers that can process information, have memory, and learn autonomously like the human brain. Among them, an important development direction of neuromorphic computing networks is hardware neural networks based on memristive synaptic devices, and Nanowire networks (NWNs) developed in recent years are a unique representative.
Recently, scientists from the University of Sydney, Japan's National Institute of Materials Science (NIMS) and their collaborators have achieved breakthroughs in this field. They proposed the neuromorphic dynamics of a network of artificial nanowires. Through simulations, they found that when subjected to electrical stimulation, this network of nanowires adjusts in a brain-like manner.
The research team also found that by keeping the nanowire network in a state similar to that of the brain at the “edge-of-chaos” state, it was proved that the system can perform tasks at the optimal level. This discovery opened up the development of artificial intelligence. An exciting new path.
The research was titled "Avalanches and edge-of-chaos learning in neuromorphic nanowire networks" and was published in Nature Communications on June 29.
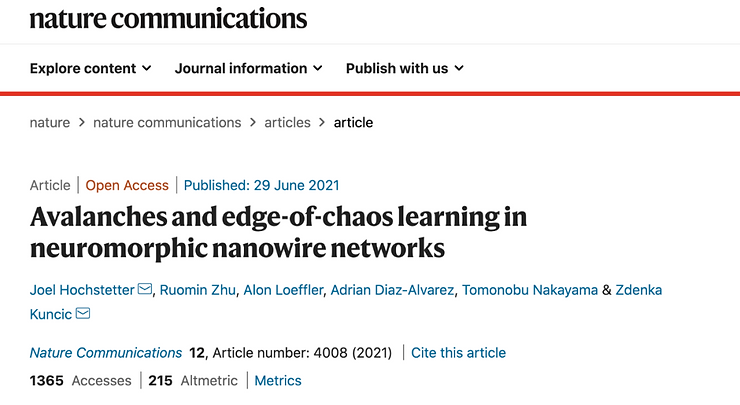
Joel Hochstetter, one of the authors of the study and a Ph.D. student at the University of Sydney’s Nano Research Institute and School of Physics, said: “On a two-dimensional plane, we randomly arrange wires 10 microns long and no more than 500 nanometers thick. Where the wires overlap In place, an electrochemical node will be formed, just like a synapse between neurons. We have found that the electrical signals passing through this network will automatically find the best path to transmit information, and this architecture allows the network to "remember" the previous path through the system."
"Edge of Chaos" opens up a new path for artificial intelligence
In recent years, an international research team composed of Professor Zdenka Kuncic and his Ph.D. student Joel Hochstetter from the University of Sydney and Professor Tomonobu Nakayama from Japan’s National Institute for Material Science (NIMS) has worked in the Nano A lot of research has been done in the research field of a wired network, including the dynamic behavior of nanowire network, network topology and so on.
The term edge of chaos was coined by mathematician Doyne Farmer to describe the transition phenomenon discovered by computer scientist Christopher Langton. The edge of chaos initially refers to the interval of the variable λ, in which the behavior of the cellular automata (CA) is observed to change. As λ changes, the behavior of cellular automata undergoes a phase change.
It is generally believed that in a nonlinear dynamic system, optimal information processing can be achieved when the phase change (the so-called critical point) is approached. Different phase transitions are related to criticality, especially "avalanche criticality" and "chaotic edge criticality".
In the critical state of avalanche, the system is at the critical point of the transition of activity-propagation, and the disturbance of the system may cause cascades within a certain scale and duration, which is characterized by a scale-free power-law distribution; in the critical state of chaos edge, The dynamic state of the system is between order and disorder, and the system keeps infinite memory of disturbance. Chaotic edge dynamics have been observed in cortical networks and seem to optimize the computational performance of recurrent neural networks, echo state networks, and random Boolean networks.
The nanowire network is embedded with a top-down nanomanufacturing method. This self-assembly method gives a complex structure to its network circuit, which can achieve higher interconnectivity of the resistance switch memory (memristive) cross-point junction. Regardless of the structural or functional relationship, the nanowire network has a strong similarity with the real (biological) neural network formed by bottom-up self-assembly.
The neuromorphic nanowire network can be used for a series of information processing tasks. Information can be stored in memory node pathways for static tasks such as associative memory and image classification. To gain a deeper understanding of the neuromorphic dynamics of nanowire networks, in this study, Hochstetter and his team designed a physically-driven Ag-PVP nanowire network computational model.
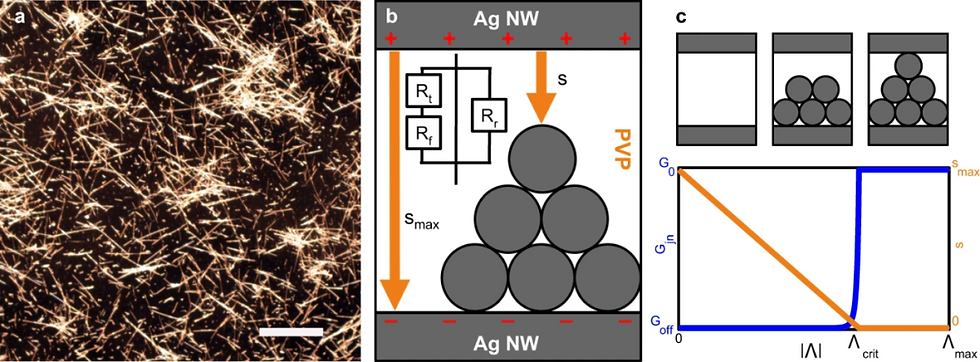
Figure | Ag-PVP nanowire network and memory junction (Source: Nature Communications)
Ag∣PVP∣Ag junctions are located at the intersections between nanowires. These electrical junctions are modeled as voltage-controlled memristors. By adjusting the state of the conductive silver wires in the junction of the PVP layer, the electrical conductivity of the junction can be controlled. Researchers use the model to simulate and analyze the network-level dynamics of the neuromorphic system to understand how to make it perform best when solving simple tasks.
Research has found that if the signal stimulating the network is too low, the path will be excessively predictable and orderly, and will not produce a sufficiently complex output; if the electrical signal floods the network, the output will be completely chaotic and useless to solve the problem. The best signal that produces a useful output is exactly on the edge of this chaotic state.
In this regard, Kunic said: “Some theories in neuroscience show that human thinking can operate at the edge of chaos or in a so-called critical state. Some neuroscientists believe that it is in this state that our brain can reach the most comprehensive level. Performance."
"The exciting result of this result is that it shows that these types of nanowire networks can be adjusted to have a diverse, brain-like collective dynamic mechanism that can be used to optimize information processing." Kuncic said.
Due to the coupling of the reminiscence node and the recursive network topology, the nanowire network becomes an ideal choice for time information processing when implemented in the energy storage calculation framework, such as signal conversion and nonlinear time series prediction. The researchers pointed out in the paper that these applications indicate that the nanowire network is a promising neuromorphic system that can be used for adaptive signal processing of streaming data.
Overcoming the duality of computers
In the nanowire network, the connection between the wires allows the system to combine memory and computing into one system, which is different from a standard computer, which separates the memory (RAM) and the central processing unit (CPU). The neuromorphic network is formed by the random self-assembly of silver nanowires. The silver nanowires are coated with a layer of the polymer after synthesis. The junction between the two nanowires acts as a resistance switch, just like in a biological neural network. Synapses of the nerves.
In this regard, Hochstetter said: "These nodes act like computer transistors, but have an additional feature, which is to remember the path the signal has traveled before. Therefore, they are called memristors."
This kind of memory takes a physical form, that is, the cross-connect points between nanowires are like switches, and their behavior depends on the historical response to electrical signals. When a signal is applied to these nodes, tiny silver wires will gradually activate these nodes by allowing the current to flow. "This creates a memory network in the random system of nanowires," Hochstetter said.
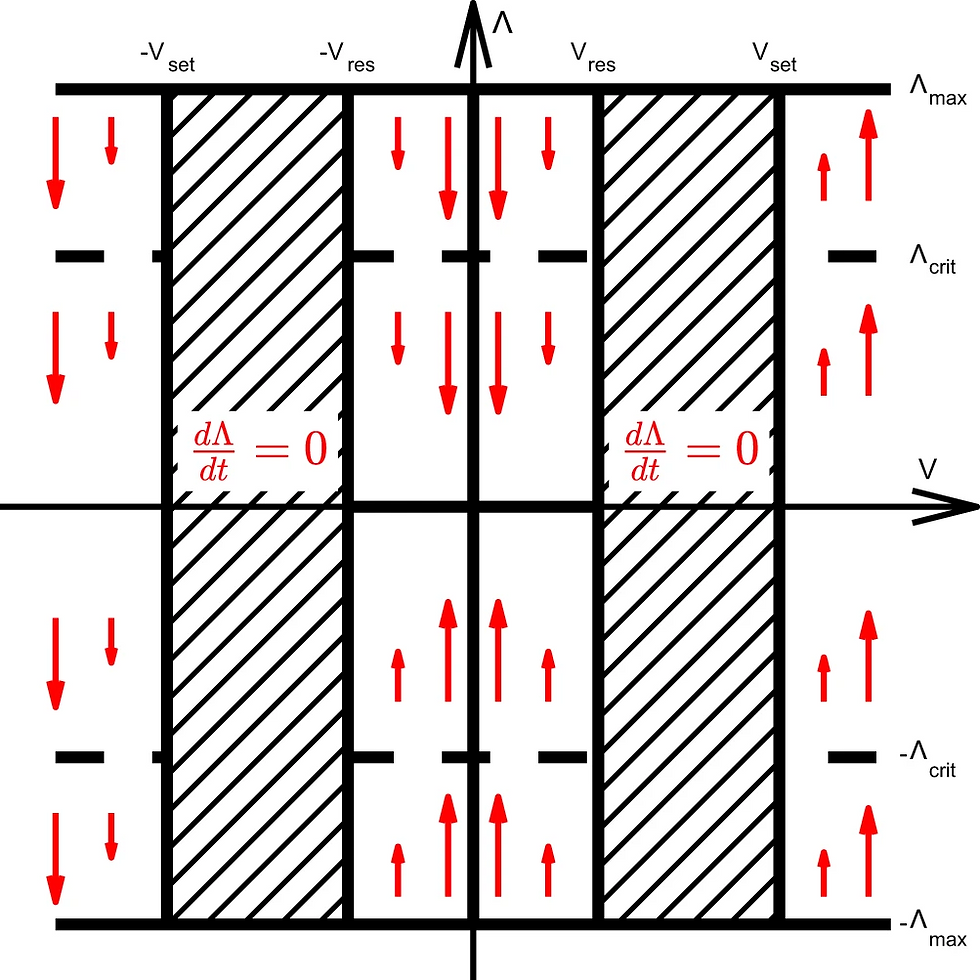
(Source: Nature Communications) Figure | Illustration of the filament formation model (the horizontal axis represents the junction voltage, and the vertical axis represents the function of the junction voltage in the threshold memristor junction model)
Based on this, Hochstetter and his team built a simulation of a physical network to show how to train it to solve very simple tasks. They trained the network to convert a simple waveform into a more complex waveform type.
In the simulation, they adjusted the amplitude and frequency of the electrical signal to see where the best performance occurred. "We found that if you push the signal too slowly, the network will do the same thing over and over again without learning and development; if you push it too fast, the network will become unstable and inaccessible. Forecast." Hochstetter said.
Professor Kuncic believes that the combination of memory and computing has huge practical advantages for the future development of artificial intelligence. "The algorithm that needs to train the network knows which node should be assigned the appropriate ‘load’ or information weight, which consumes a lot of energy."
"The system we are developing does not require such an algorithm. We just allow the network to develop its own weights, which means that we only need to consider the input and output of the signal. This framework is called reservoir computing. The network weight is adaptive and can release a lot of energy." Kunic said, which means that any artificial intelligence system using this network in the future will achieve lower energy consumption.
The development prospects of nanowire networks
In fact, in a review titled "Neuromorphic nanowire networks: principles, progress and prospects for neuro-inspired information processing" published in Advances in Physics: X this year, Kunic, Hochstetter and others have already commented on nanowire networks. The future development direction of the company has been portrayed.
They stated that the memory properties of the metal-core nanowire network have been widely described, but more research is needed to clarify the key role played by its complex and disordered network structure, which has a great impact on the nonlinear dynamics that appear. Big impact. Kuncic believes that expanding knowledge and technology from the fields of complex physical systems, statistical physics, nonlinear dynamics and network neuroscience, as well as reservoir calculations may make significant progress in the research field of emerging artificial neural network models.
The paper points out that organic nanowires or nanofibers with conductive properties grown through bottom-up self-assembly are also worthy of further study. Comparing the information processing capabilities of organic and inorganic nanowire networks may reveal biological mimicry in neuromorphic systems. And new insights into the importance of biological credibility.
In addition, another future research direction of the nanowire system is its potential in neuromorphic quantum information processing. So far, research on neuromorphic quantum computing has focused on encoding artificial neural networks into quantum computing hardware systems. However, neural networks can be sandwiched in quantum hardware using superconducting nanowires to realize a neuromorphic system capable of quantum information processing.
So, how to go beyond the existing machine learning benchmark tasks and use nanowire networks for neuromorphic information processing? The researchers pointed out that future efforts should focus on complex signal processing tasks and the classification of streaming space and background information under the constraints of preserving causality. To achieve true neuromorphic intelligence, there is still a long way to go.
Reference
https://www.nature.com/articles/s41467-021-24260-z
https://www.eurekalert.org/pub_releases/2021-06/uos-oc062721.php
https://www.tandfonline.com/doi/full/10.1080/23746149.2021.1894234
https://ieeexplore.ieee.org/document/9181034
AI
Technology
Future
Artificial Intelligence
Machine Learning
Comentários